Real-time Quality Inspection Matters, and Here’s Why
- mikemohseni70
- Mar 24, 2021
- 4 min read
Updated: May 19, 2021
By Mike Mohseni
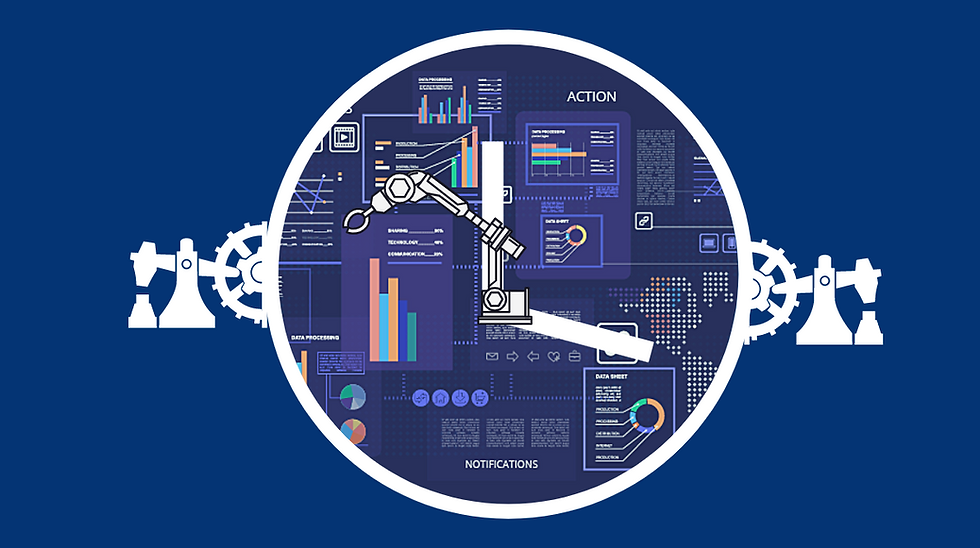
What is real-time quality inspection?
In mission-critical manufacturing operations that involve materials processing, defects can potentially lead to disasters, such as welding defects in the body of a bridge structure. In these operations, quality inspection is critical. The manufacturing industry adopts various solutions to ensure quality, and intensive post-process inspection is the most used approach.
What are the benefits?
The risks and costs associated with post-process inspections are still causing significant downtime and financial losses in the manufacturing sector. The cost of addressing defects sharply increases as the defective parts move up in the manufacturing cycle. The time required for reworks is a major contributor to the estimated 4 to 20% of global manufacturing capacity loss due to downtime. Nevertheless, real-time quality inspection of materials processing operations is costly and challenging.
The complex nature of materials processing operations such as industrial welding often requires real-time inspections to be done manually, which is costly, time and labor-intensive, and error-prone. Furthermore, it is rarely possible to inspect every part, which provides a fractional view of the manufacturing line. For example, in the automotive supplier industry, quality control guidelines instruct inspecting only between 2 – 10% of the production line.
Given the importance of reducing costs and improving efficiency in a $13.17T market, the manufacturing sector has adopted different approaches to avoid the costs of poor quality (CoPQ).
What are the alternatives?
1 – One of the most common approaches, possibly due to its low capital cost requirement, is process optimization and adjustments based on lagging metrics such as First Pass Yield (FPY), Material Yield Variance (MYV), Overall Equipment Effectiveness (OEE), and Avoided Cost. More recently, AI-powered management software solutions have facilitated the tracking and even prediction of these metrics. It is essential to evaluate the efficiency and productivity of manufacturing facilities by tracking performance metrics. However, these metrics may highlight process-level defects only after major costs have been incurred. The highest risk is considering a large error margin due to materials processing defects as an acceptable part of calculating efficiency metrics and not seeking process-level improvements.
2 – The progress in Industrial Internet of Things (IIoT) technology and its adoption have created notable opportunities to enhance manufacturing quality. Multiple sources have estimated that improvements in manufacturing operations driven by IoT applications can generate hundreds of billions of dollars in annual economic value.
In product quality control, IIoT systems provide indirect indications based on monitoring the environmental or machine conditions. Machine condition parameters such as speed and vibration and environmental parameters such as temperature and humidity are monitored to identify when they go beyond the normal thresholds. This approach provides simple binary classification, whether the product is a “pass” or “fail” and is intrinsically less accurate than evaluating process-level quality indicators. More importantly, in complex operations such as materials processing, detailed knowledge of the physics of the process, influencing parameters, and their interconnectivity with equipment are required to correlate ambient or machine conditions with ultimate product quality.
3 – The costliest approach to enhance materials processing quality is the renewal of operation modules. The financial and time costs of replacing manufacturing machinery may not be affordable to all manufacturing plants, especially SMEs.
Employing process automation and robotic systems in manufacturing lines enhances the execution and, ultimately, product quality. Despite the benefits, quality inspection still follows the manual and post-process path due to significant variabilities in materials processing, the high number of process parameters, and the complexity of the robotic systems.
More recently, companies such as GM have incorporated inline robotic quality inspection systems. The robotic arms equipped with vision and laser systems are programmed to inspect the part quality based on visual and geometrical features. This approach enhances the accuracy of executing inspection processes relative to manual methods. In addition to the capital cost requirements, the pain points such as post-process inspection, partial line inspection, and downtime due to a serial operation remain unaddressed.
4 – Modular systems for process monitoring and defect detection are viable alternatives in comparison to inspection robotic arms. Various technologies are available for process monitoring such as X-ray, ultrasound, laser, and optical systems. The last two are the most used in industrial lines considering the ease of deployment, analysis time, and low hardware sensitivity. The inline use of monitoring systems enhances safety due to remote monitoring. The recorded track of data is an important means for manufacturers to avoid liability issues.
The quality inspection based on monitoring tools remains manual and inherits the issues of being error-prone, labor-intensive, and often, post-process as the process data is reviewed only when nonconformity reports raise concern.
Integration of advanced learning-based algorithms such as deep learning with monitoring hardware enables automated defect detection systems to distinguish defective parts in the manufacturing line, log, and notify operators. High changeover rates, the variability of type and morphology of defects, and the complexity of the materials processing operations in manufacturing lines significantly limit the application of automated defect detection systems.
Opportunities
The reports from industries such as food processing and electronics packaging show the promising benefits of an AI-powered automated, real-time quality inspection system. In these industries, high changeover rates and variabilities are not large issues compared to in the welding line of an automotive supplier plant. However, the resulting cost savings and production efficiency improvements show the great opportunity and the potential future path for quality enhancement systems in welding and generally material processing applications.
Comments